When Connie Lehman, professor of radiology at Harvard Medical School and a radiation specialist at Massachusetts General Hospital, started working on computer-aided design (CAD) back in 1998, she was excited about its potential for improving the detection of breast cancer. She was convinced that CAD technology would help radiologists find more cancers, lead to earlier diagnoses, and potentially produce higher cure rates.
But the story didn't end the way she thought it would. "Although studies in the lab found that CAD could make a difference, it wasn't having the impact we were hoping for in the clinic," she recalls. "But I'm cautiously optimistic that the new AI [artificial intelligence] models will be more successful in leveraging the power of computers to enhance the impact of imaging."
Much research is under way to validate the accuracy of artificial intelligence models for detecting breast cancer, especially from mammograms. Some studies are investigating the overall accuracy of AI relative to readings done by radiologists; others are looking at its ability to avoid false positives. Work is also being done to determine whether AI can predict future cancer risk. The results have been encouraging, paving the way for greater clinical use of AI.
Much research is under way to validate the accuracy of artificial intelligence models for detecting breast cancer
What has changed over the past 26 years? Krzysztof Geras, assistant professor of computer science at New York University, explains that "we have better machinery, and importantly, we have developed deep learning, a subset of AI, that gives models the capacity to learn from large amounts of data."
The models, he adds, can learn from images and can operate much faster than humans. "The more sophisticated the model, the better it can be at pinpointing patterns and making predictions—even about the onset of cancer.”
Multiple Uses of AI
Numerous studies have verified the value of AI in reading mammograms and detecting cancer. For example, in a randomized controlled study published in the August 2023 issue of Lancet Oncology, researchers in Sweden divided women into two equal groups. From the first, about 40,000 mammograms were analyzed using only AI. From the second, two radiologists reviewed about the same number of mammograms.
Cancer detection rates were slightly higher in the AI-only group (244) than in the two-radiologist group (203). That AI performed better, and had the added benefit of a reduced workload for radiologists, points to the value of continued research of this approach—and its potential for more use in clinics.
Radiologists could also rely on AI to identify low-risk mammograms, allowing them more time to focus on more high-risk cases. In a study published in the journal Radiology: Artificial Intelligence on April 10, 2024, researchers from Washington University School of Medicine in St. Louis and Whiterabbit.ai, an AI company, found that AI had read negative mammograms correctly, reducing the number of false positives and improving the workflow of the radiology team. The ability of AI to triage mammograms by level of risk is an advantage that Stuart Kaplan, section chief of breast imaging at Mount Sinai Hospital in Miami, is particularly interested in as he considers adding AI to his team's arsenal of tools.
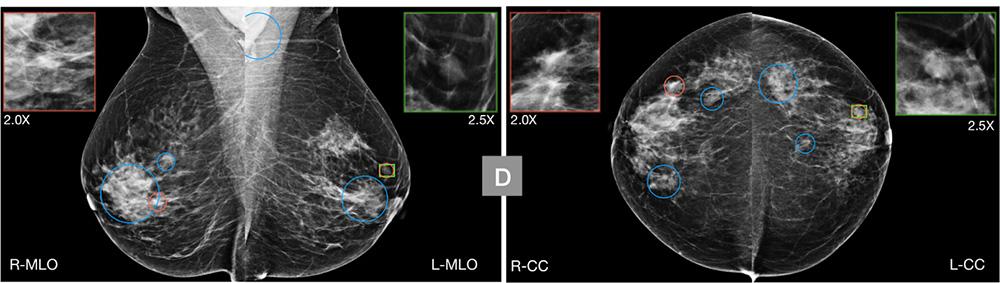
For many researchers in the field, however, the potential of AI to detect breast cancer before it develops is what has sparked the most excitement. Lehman and her partner, Regina Barzilay, a professor at MIT, have collaborated on one of these models, which they have named Mirai.
For Barzilay, the work is personal. Ten years ago, she was diagnosed with breast cancer and went through extensive treatment. The experience made her wonder whether artificial intelligence could help diagnose tumors earlier so that treatment could be less invasive. At the time, she was studying another aspect of AI, but decided to switch fields to try to answer this question.
She and Lehman have had a fruitful partnership. One of the high points was the publication of their study testing the accuracy of Mirai in the Journal of Clinical Oncology in November 2021. Using 128,793 mammograms from 62,185 patients across seven sites, both in the United States and abroad, Mirai correctly identified an average of about 76 of 100 cases, a significant improvement over other risk models. What's more, Mirai was consistent across countries and in both white and Black populations.
What is the secret behind Mirai's success? Interestingly, researchers are unsure about how AI does it.
"We know that AI works, but we're not always clear about the mechanisms it uses," says Lehman. "This phenomenon is known as the black box of AI, and it refers to characteristics that may be significant but that the human eye can't see."
Gervas agrees, adding, "we understand how deep learning works and how the model learns. What we don't understand is exactly what the model is looking for in the data. It's probably learning from visual markers not known to humans. Furthermore, the system can learn from data relentlessly and is super fast. The human visual system is not adjusted to this kind of learning."
Next Steps for AI and Breast Cancer
Researchers are forging ahead with new studies, modifying known models and trying new approaches. For example, a group of researchers from Duke University addressed the concerns about Mirai head on. They simplified the system, focusing on only one characteristic—breast asymmetry. A study published in Radiology on March 19, 2024, reported that its system, called AsymMirai, performed almost as well as Mirai in predicting whether someone will develop breast cancer within 1 to 5 years based on this one characteristic. The data came from 210,000 mammograms from 81,824 patients. Further, the study confirmed asymmetry as an important imaging marker for breast cancer risk.
The experience made her wonder whether artificial intelligence could help diagnose tumors earlier so that treatment could be less invasive
AI also has been used to read data from ultrasounds and MRI images. After extensive training, the systems can make predictions from this data as well. One study found that AI is able to reduce the number of false positives from ultrasound images.
"At this point, I think that AI is accurate enough to read any kind of data and provide solid predictions," Gervas says. "What I think would be really powerful is to collect data from all three modalities [mammography, ultrasound, and MRI] on each individual and use the combined information to predict cancer risk. That would be the most effective way of all to use AI."
Lehman also believes that AI can be used in multiple ways. She's pleased that new models are beginning to incorporate images from Asian, Hispanic, and Black women, a strategy to address racial inequities in breast cancer diagnosis and treatment. This trend will likely continue in the future. It will also be possible to partner with the genomics community and discover new ways to work with women with mutations that are known to lead to breast cancer.
"We are in the midst of a revolution in AI," Lehman says. "I don't want to let this opportunity slip away. It could have a huge impact on how we treat patients."