There is a well-known tale of a thirteenth-century Sufi philosopher named Nasreddin who lived in what would be present-day Turkey. In this story, Nasreddin enlists a friend to help him find his lost keys late one night. They search near a lit lamppost in the street for over an hour. Finally, the friend asks, “Are you sure you lost your keys here?” Nasreddin points to a nearby area shrouded in the darkness and says, “No, I lost them over there, but the light here is better.”
I lost them over there, but the light here is better.
—Nasreddin
In 2019, the Nobel Prize for Economics was awarded to Abhijit Banerjee, Esther Duflo, and Michael Kremer for “their experimental approach to alleviating poverty.” There are numerous examples of the positive impact of their work and that of their associates, which utilizes experimental approaches developed for biostatistics and clinical science to answer specific development policy questions. In one study, Kremer and Edward Miguel showed that charging people money for deworming drugs (i.e., user fees) dramatically decreased uptake of the intervention and hence lessened the efficacy of deworming programs. In another study, two authors affiliated with the laureates—Jessica Cohen and Pascaline Dupas—showed that even nominal fees charged for bed nets were a significant barrier to the poor accessing and using the nets for malaria prevention. Cohen and Dupas randomized the price of the bed nets across intervention and control groups and diligently tracked outcomes. The results of this experiment provided the evidence needed to push back against the prevailing wisdom of the time—that unless the poor paid something for these commodities they would not value them, and hence the bed nets would remain unused.
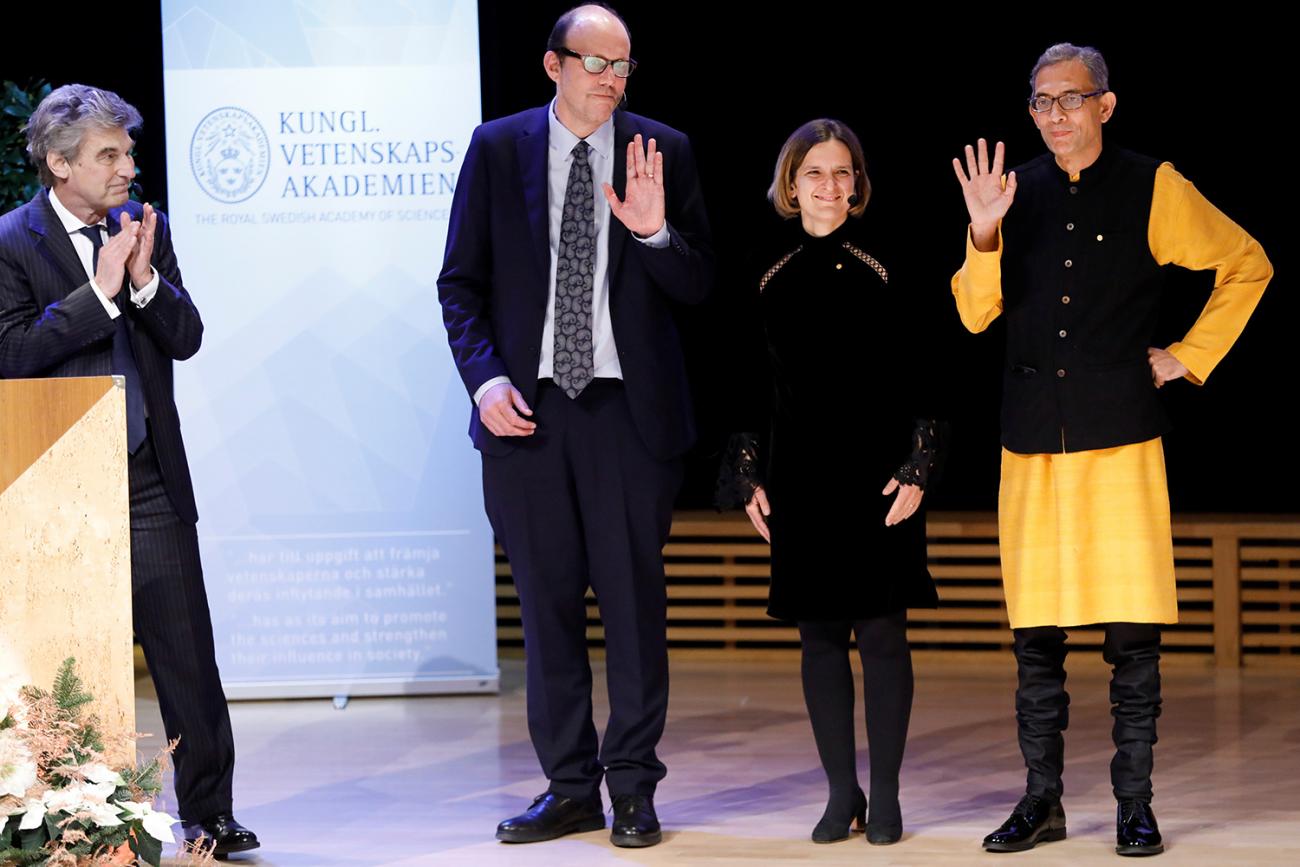
Banerjee, Duflo, and Kremer have taken on many such issues by breaking down complex policy topics into bite-sized questions that can be studied through experiment. Their research has provided clarity on questions ranging from school absenteeism to farm subsidies for fertilizer.
Without guidance, policymakers are forced to act without scientific input or, worse, take no action at all
The Nobel Prize committee noted without exaggeration that the laureates’ “experimental approach now dominates development economics.” Meanwhile, experimental approaches increasingly dominate research in public health, education, and other fields. A challenge arises, however, when certain topics are not amenable to exploration through experimental design. Research and funding increasingly prioritize approaches that are backed by evidence and can demonstrate a clear impact. But what about the questions that remain “in the dark,” fraught with uncertainty? Searching and acting only in the lit space ignores the structural, institutional, and systemic challenges that impede progress. Without guidance, policymakers are forced to act without scientific input or, worse, take no action at all.
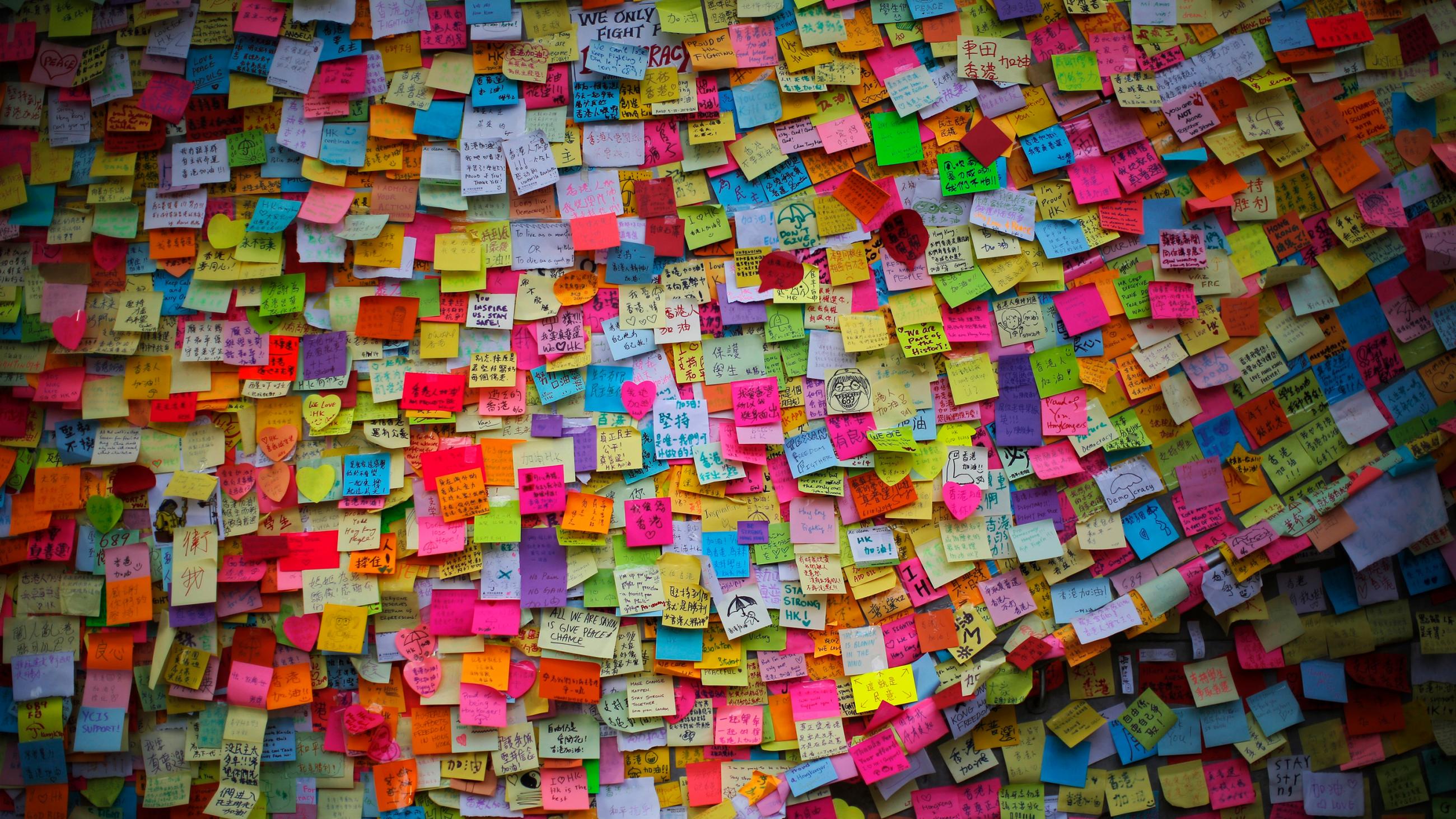
Evidence Grading Standards and the Need to Grade on a Curve
Evidence is often used to guide international development and public health spending— focusing on what works while defunding what does not. Karl Popper, a renowned twentieth century philosopher of science, argued that for something to be considered “science” it had to make testable predictions that could be proven false. Astrology is not a science because its predictions are too nebulous, the influence of planets too subtle, and its interpretations too open-ended to be tested and proven right or wrong. By bringing scientific methodology (i.e., experimental design) to global public health, the laureates have made decision-making more scientific and less beset by politics, biases, and other confounding variables.
Alongside the rise of experimental methodology, evidence grading approaches have been developed to rate the quality of evidence, recognizing that it is impossible for anyone to read and evaluate the volume of new original studies. Multiple groups including the University of Michigan, the Agency for Healthcare Research and Quality (AHRQ), the Oxford Center for Evidence-Based Medicine (CEBM), and Cochrane have all proposed evidence grading guidelines. These are described briefly in the table below, and sources for the four approaches are here, here [PDF], here, and here.
Most grading guidelines require multiple randomized controlled trials that support the benefits of intervention in order for the evidence for the intervention to be considered “strong.” These grading systems usually label studies that rely on “expert opinion” alone as “weak” or “very low”—even when those experts are in agreement. This approach has merit as sometimes, as with the cost-sharing experiments described above, experts can be in agreement and still be wrong.
However, these approaches to rating evidence fail to recognize that some critical questions could be more difficult, or in some cases impossible, to study through experimental design. Experimental research can be difficult to carry out due to:
- the nature of the intervention;
- how “distal” or removed the intervention is from the outcomes;
- challenges in measuring the outcome of interest, particularly when many outcomes are related to the intervention;
- ethical challenges in conducting the experiment; or
- the political acceptability of research using experimental methods when the public already believes in the intervention.

In effect, research can be graded as “very low” or “weak” as a result of two distinct scenarios:
- instances in which using experimental methods to better study the issue would be relatively easy, but such studies have not yet been done; and
- instances in which the research question could be hard, or even impossible given political or ethical realities, to study through experimental design, despite being important to answer.
The term minimally important clinical change recognizes that experimental evidence for an intervention can exist without it being worth recommending
Whether intended or not, interventions designated as having a “very low” or “weak” evidence base have a harder time attracting resources compared with approaches for which the evidence is “very strong.” Yet the importance of an intervention could have nothing to do with the level of evidence for it. Conversely, an intervention can be supported by a strong level of evidence, randomized controlled trials showing statistical significant differences between treatment and control groups, yet still have minimal impact. For example, statistically significant improvements on self-reported pain scales when taking supplements such as chondroitin sulfate led the authors of one randomized trial to conclude that chondroitin sulfate “improves hand pain and function.” But it is not clear what a change on this scale means in terms of people’s lives. Such results have led other authors to attempt to coin the term minimally important clinical change, recognizing that experimental evidence for an intervention can exist without it being worth funding or recommending.
The strengthening of health systems is an example of an approach that has been under-prioritized by virtue of being hard to study. For decades, advocates have bemoaned the neglect of health systems and capacity building as donors focused on vertical programs, which has been defined as having targeted “objectives, usually quantitative, and relating to a single condition or small group of health problems”. Admittedly, studying and quantifying the effects of a specific program such as vitamin A supplementation using a randomized experimental design is far easier than conducting a systems strengthening investigation with a national ministry of health, in which the policy context is complex, the outcomes are heterogeneous, and the payoffs occur over a long time period.
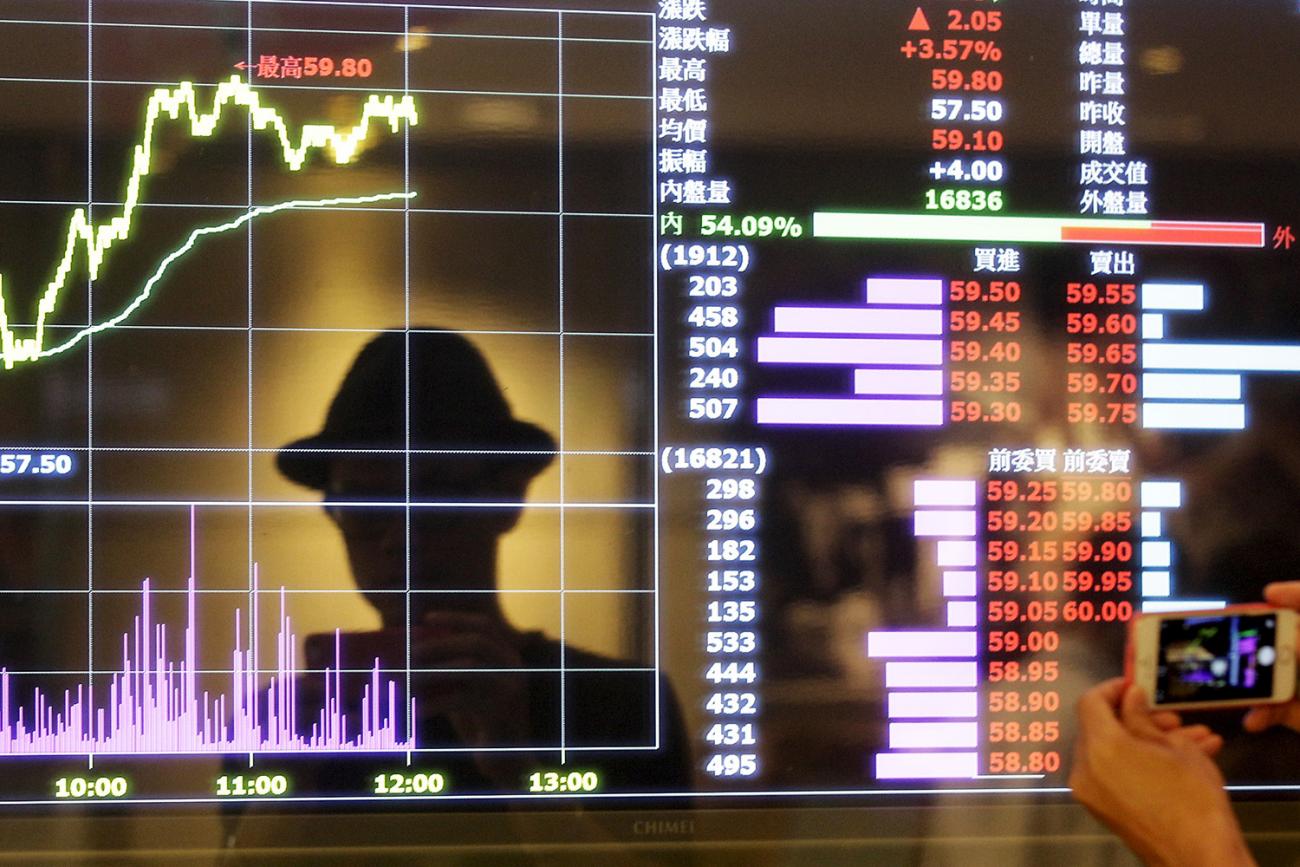
Health financing, one of the six essential health system building blocks, appears to be another topic that could suffer from experimental approaches to learning. The field focuses on a system’s approach to resource mobilization and allocation to deliver quality health services without resulting in individual financial hardship. In 2017, Cochrane published its analysis of financing arrangements for health systems in low-income countries, examining 15 systematic reviews comprising 276 individual studies. The broad questions included:
- how to raise funds to pay for services;
- the effects of different insurance schemes;
- financial incentives for health workers; and
- financial incentives for beneficiaries.
This lack of clarity and evidence could lead some policymakers and donors to conclude resources are best allocated elsewhere
As expected, the evidence for each approach reviewed ranged from “very low certainty” to “low certainty” with only a couple subtopics rising to the level of “moderate certainty.” The authors “identified gaps in primary research because of uncertainty about applicability of the evidence to low-income countries.” They concluded that “the effects are uncertain” for donor assistance, caps and co-payments, pay-for-performance schemes, and provider incentives. In other words, the review was unable to provide any guidance for policymakers designing health financing approaches. What limited evidence that did exist, according to the review, did not make the grade. This lack of clarity and evidence could lead some policymakers and donors to conclude resources are best allocated elsewhere.
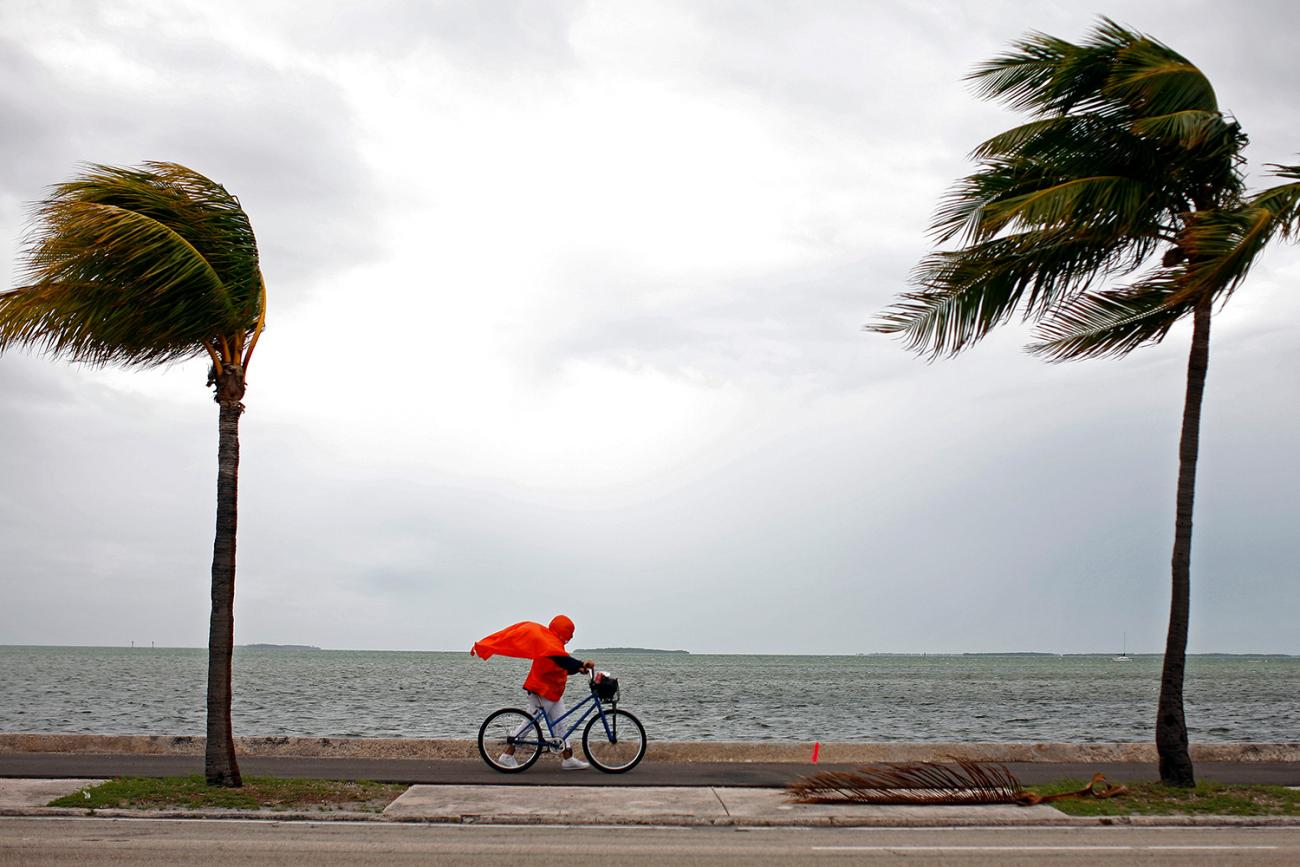
Learning From Other Fields
Many fields do not rely on randomized experimental design as the primary means of knowledge gathering. Anthropology, sociology, and political science all have their own approaches to evaluation and learning, though the rise of the randomized trial has impacted these disciplines as well. Climate science, meanwhile, has developed into a rigorous field without requiring randomized intervention and control groups. After all, there can be no control group when we have only one planet Earth. The field instead utilizes simulation models that combine evidence derived from atmospheric chemistry, physics, and even policy scenarios. Although the sensitivity of various model parameters are discussed within the field ad nauseam, few suggest that the lack of randomization invalidates its results and strength of evidence.
Climate science utilizes simulation models that combine evidence derived from atmospheric chemistry, physics, and even policy scenarios
The current COVID-19 crisis also highlights numerous important questions that have to be answered without the benefit of experimental studies, much less systematic reviews of multiple such studies. When to close schools and for how long? The effect of school closures on transmission of the virus is a critical question, yet there are at best a limited number of natural experiments to look toward for guidance. These studies will likely never make the “grade” to constitute strong evidence, as it is impossible to determine whether the decision to close schools took place in isolation of confounding variables. Importantly, well-known structured techniques exist for assessing expert opinions and consensus in a way that serves to limit bias. These approaches have to rise to the occasion to answer difficult questions, as the results are better than avoiding such questions altogether and sidestepping the need to provide critical guidance.
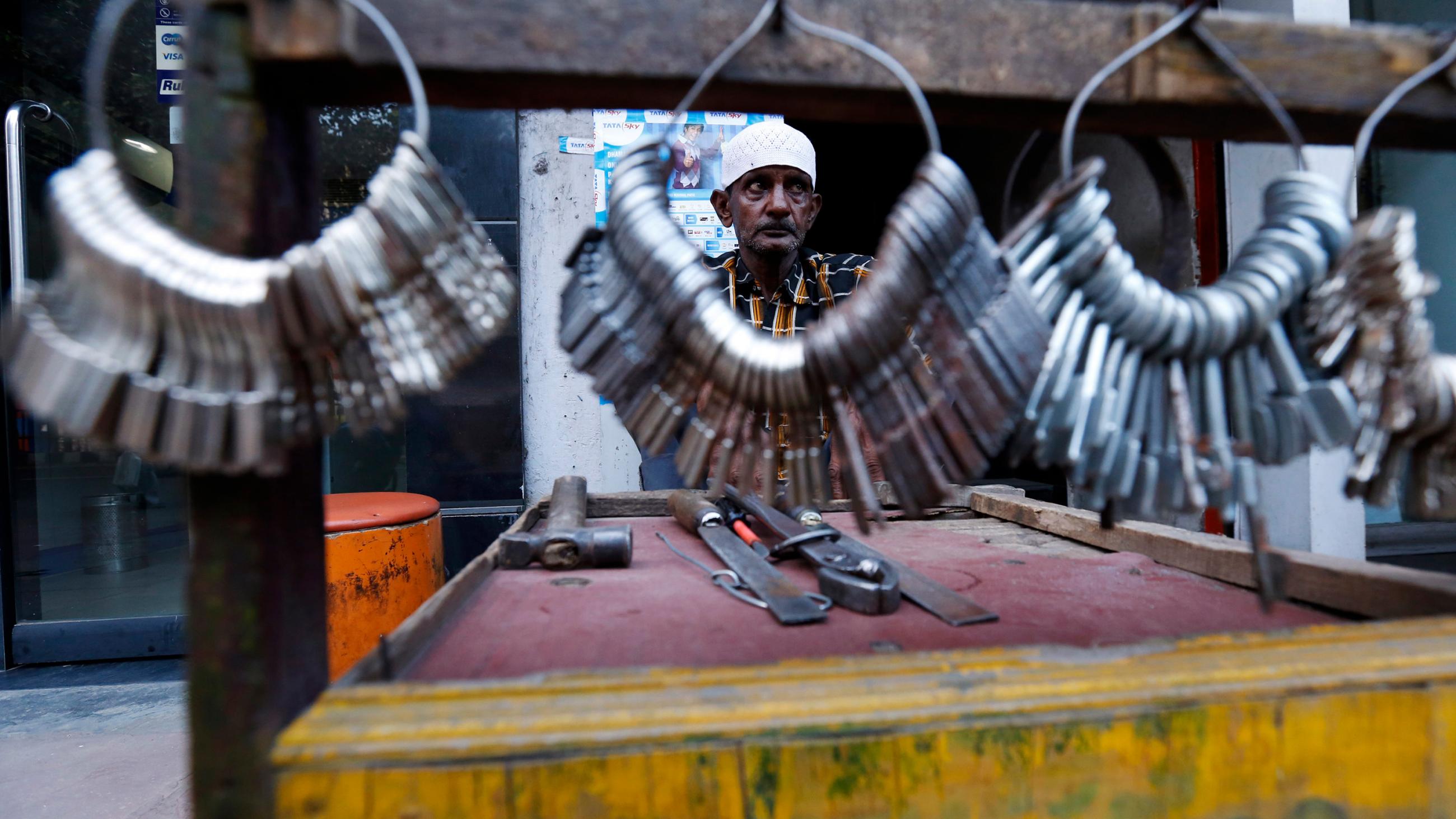
A recent review of health financing not bound by evidence grading standards, for example, demonstrated broad consensus to direct future approaches toward the field even while indicating areas in which evidence was limited. The review provided concrete guidance for countries and donor agencies alike, charting a course for progress toward universal health coverage. Recommendations focused on resource mobilization, quality improvement, efficiency, and equity to improve health outcomes and reduce financial hardship associated with out-of-pocket payments. Nevertheless, these sorts of reviews often do not find the same marquee placement in prestigious academic journals as those based on experiment.
These sorts of reviews often do not find the same marquee placement in prestigious academic journals as those based on experiment
If the actions of the Nobel Prize committee serve as a zeitgeist within the field, the decision to award Banerjee, Duflo, and Kremer reflects the current orthodoxy that views randomized experiment as the true arbiter of evidence and knowledge. Yet for the foreseeable future important questions will arise for which there is no such evidence to rely on. This could be because the problems are hard to study or because the situation is novel with an urgent need to take action. In these instances, characterizing the evidence that exists as “weak” or “low” could underestimate what is actually known about the topic. As a result, policymakers could underinvest in what is needed, choosing instead to invest in other areas with “strong” evidence but weak impacts.
To apply our Sufi parable to the global health challenges of the day, the reality is many “keys” on the ground can be used to inform programs and policies. Some of these shine bright under the lights of experimental design, and for these keys randomization methodology should continue to both confirm knowledge and overturn cherished but false beliefs. But then there are the keys lying in the darkness, keys that researchers cannot ignore by keeping within the field of light.